Cognitive K.i. Empowering AI Solutions for Professionals in Diverse Fields
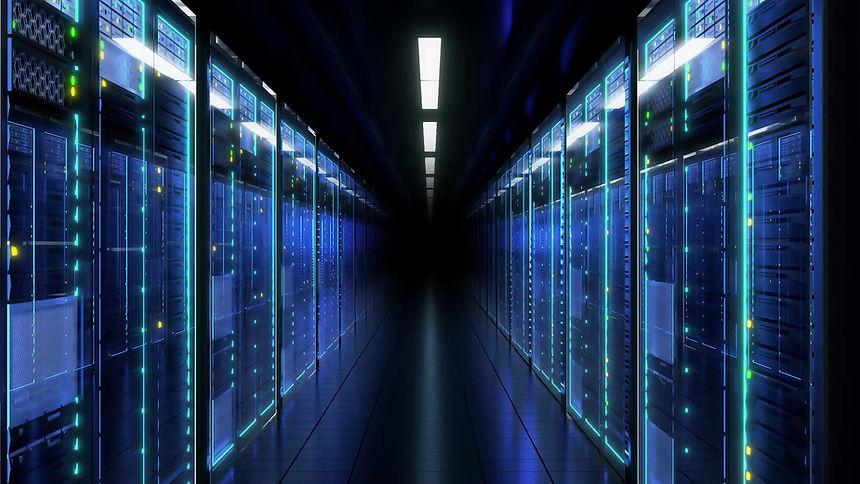
Sentiment Analysis
A sentiment analysis model is a type of natural language processing (NLP) and machine learning model designed to analyze text and determine the emotional tone or sentiment expressed, classifying it as positive, negative, or neutral.
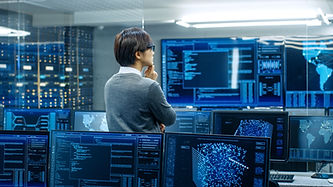
Sentiment Analysis
k.i. - Sentiment Analysis
Sentiment analysis is a critical component of Cognitive K.i. It plays a significant role in understanding the emotional tone behind a series of words. It leverages natural language processing (NLP) to systematically identify, extract, and quantify subjective information from textual data. This capability has become increasingly vital in various domains, particularly in tracking changes in market dynamics and enhancing data analytics.
In the financial sector, sentiment analysis offers a novel approach to gauge public perception regarding companies, stocks, or trends, often accurately predicting market movements. By analyzing social media posts, news articles, and financial reports, AI algorithms can discern the prevailing positive, negative, or neutral sentiment associated with a particular entity or event. A surge in positive sentiment regarding a tech company may precede an uptick in stock prices, while negative sentiment could foreshadow declines or heightened volatility. This predictive capability is invaluable for investors and financial analysts seeking to inform their strategies based on market sentiment rather than solely on quantitative metrics.
Sentiment analysis extends beyond finance, impacting the retail, tourism, and public health sectors. Businesses utilize sentiment assessment to understand customer feedback, enabling them to adapt their offerings to align with consumer expectations. This approach improves products and services, fostering customer loyalty and enhancing brand reputation. In public health, sentiment analysis can track public perception of health campaigns, providing insights that lead to more effective communication strategies.
The advancements in machine learning and deep learning have significantly improved the accuracy of sentiment analysis tools. Techniques such as recurrent neural networks (RNNs) and transformers have enabled models to comprehend context and nuance, overcoming challenges associated with sarcasm or ambiguous language. As a result, Cognitive K.i. can analyze vast amounts of unstructured data in real time, driving informed decision-making processes that reflect actual market sentiments.