Cognitive K.i. Empowering AI Solutions for Professionals in Diverse Fields
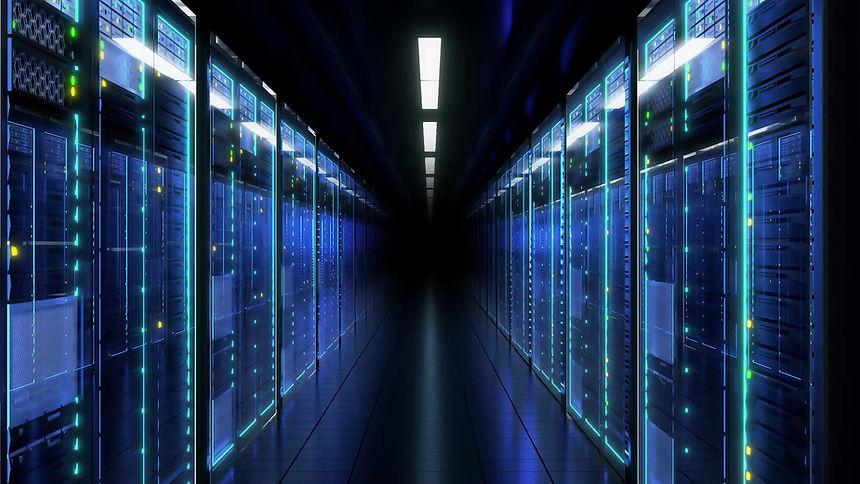
Natural Language
A multi-agent system (MAS) is a computational system composed of multiple interacting intelligent agents, each capable of autonomous decision-making, that work together to achieve complex goals or solve problems more efficiently than a single agent could
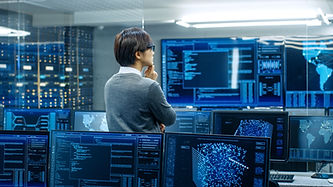
Natural Language
k.i. - Natural Language
Multi-agent systems (MAS) represent a significant paradigm within the field of artificial intelligence (AI). They are characterized by the interaction of multiple autonomous agents that work together to achieve individual or collective goals. These agents, which can be software programs, robots, or even human participants, operate within a shared environment and are designed to perceive their surroundings, make decisions, and interact with one another to solve complex problems.
At the core of a multi-agent system lies the notion of agent autonomy. Each agent possesses a degree of independence, allowing it to act based on its perceptual inputs and decision-making heuristics. These agents have specific capabilities, such as sensing, reasoning, learning, and acting upon their environments. The diversity of agents often reflects their roles; some specialize in data collection, while others focus on analysis, negotiation, or task execution. This specialization enhances the system's overall efficiency and effectiveness.
Multi-agent systems typically operate under several frameworks, which delineate how agents coordinate and communicate with one another. Standard models include cooperative, competitive, and mixed frameworks. In a collaborative model, agents work together towards a common objective, sharing information and resources. In autonomous vehicle navigation, multiple vehicles may share data about traffic conditions to optimize routing. Conversely, agents may engage in rivalry in competitive scenarios, as observed in game-theoretic contexts, such as economic markets or adversarial settings. The mixed model combines both elements, enabling agents to simultaneously collaborate and compete.
Various protocols facilitate communication among agents, enabling them to exchange information, negotiate, and resolve conflicts. Depending on the system's requirements, these protocols can vary from simple message-passing techniques to more complex negotiation frameworks. Effective communication is fundamental to the success of any MAS, as it fosters coordination and enhances the agents’ ability to adapt to dynamic environments.
The application of multi-agent systems is vast and continues to expand across different domains. MAS can optimize logistics in supply chain management by enabling various stakeholders to collaborate and negotiate. In environmental monitoring, multiple agents can collectively analyze data from distributed sensors to track ecological changes. MAS has demonstrated promise in intelligent automation, where synergistic interactions between agents result in enhanced performance and productivity in industrial settings.